DeepSea and G2 Ocean roll out groundbreaking AI voyage optimisation tool
DeepSea Technologies and G2 Ocean collaborate with SINTEF, backed by the EEA and Norway Grants 2014 – 2021 in the frame of the Business Innovation Greece Programme, to explore and develop an energy management AI solution for vessel operators using AIS and noon-report data to lower barriers to adoption.
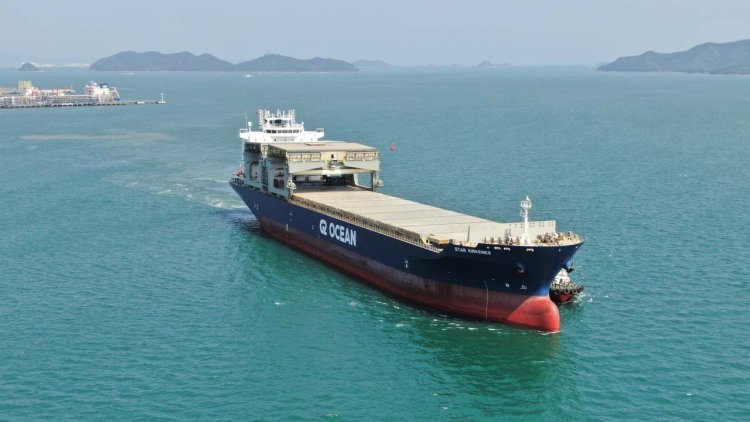
DeepSea Technologies has announced the completion of a project supported by the EEA Grants under the "Business Innovation Greece" Programme, to develop an accessible AI solution for vessel performance routing. Working with G2 Ocean and SINTEF, DeepSea has run trials to develop a novel artificial intelligence (AI) software solution which uses AIS and noon report data, together with high-frequency data from other vessels, to unlock voyage optimisation.
Improvements in operational efficiency through optimised navigation help operators to reduce fuel consumption and lower emissions. However, few companies in the shipping industry have the data collection systems in place to support data driven improvements. Together with G2 Ocean and SINTEF, DeepSea has developed an AI-based solution that can use the data available to every ship owner: AIS data and noon reports, to unlock the real benefits of performance routing. This approach is called "transfer learning" and allows high-frequency data models from hundreds of vessels already in DeepSea's databases, to be transferred over to low-frequency data vessels of the same type and further adapted to available data.
The new software solution, 'Pythia Augment', has been applied to the bulker market for the duration of this project, however, has widespread applications for all types of vessels as well, and provides route and speed guidance for individual vessels based on AI-generated energy performance modelling. DeepSea and G2 Ocean applied the solution to 5 Grieg Star vessels within the Norwegian company's fleet, to develop and pilot an AI model with the ability to produce accurate and optimised guidance that has been successfully applied to reduce fuel consumption and achieve the highest possible Time Charter Equivalent (TCE) for any given voyage. The ships used to test the solution provided an ideal training ground for developing the AI application, as the fleet features vessels operating noon reports as well as more modern live data acquisition systems.
During the project, DeepSea and G2 Ocean collaborated with SINTEF, a leading independent research organisation based in Norway to understand barriers to end-user compliance. Breaking down barriers to the adoption of AI recommendations by the crew is essential. The value of AI-driven optimisation to sustainable shipping operations can only truly be realised if there is high compliance with the recommendations produced.
Dr. Konstantinos Kyriakopoulos, Co-Founder and CEO of DeepSea Technologies commented on the project:
"Ship owners need to be taking action now to deliver on the maritime industry's long-term goal to decarbonise. A mature market for the low-carbon fuels that will drive large-scale decarbonisation of marine energy remains some way away. In the meantime, owners and operators should focus on reducing energy consumption through operational efficiencies. With the delivery of Pythia Augment using low-frequency data to optimise voyage planning, we are creating a new and innovative way for bulkers to effectively drive down carbon emissions in their operations."
Arthur English, CEO of G2 Ocean, further commented:
"The solution developed by DeepSea enables real time analysis of a range of commercial, technical, and environmental factors resulting in a reduction of emissions as well as optimised earnings. With the wide-scale adoption of green fuels still some way off, it is vital that we grasp the opportunities that are currently economically viable to reduce emissions."
DeepSea has used two critical AI technologies to unlock voyage optimisation based on legacy data: Transfer Learning and Hybrid Modelling. Having access to a fleet with mixed data acquisition systems was paramount to the development of the AI tool.